Predictive attributes
Predictive attributes are calculated in different ways depending on the attribute but they all use a combination of Machine Learning (ML) and Artificial Intelligence (AI) to predict insights or behavior. Unearth insights into your customers' future actions using patterns in their behavior over time. These insights allow you to forecast when a customer is poised to make a purchase, enabling proactive measures to boost revenue. Conversely, you can pinpoint potential prospects unlikely to convert and reduce your ad spend on these customers. The potential is limitless! You can see our list of standard predictive attributes here.
How could I use predictive attributes
Predictive attributes work just like any other attribute in the Hub. The main distinction is the logic used to develop your segments. Instead of looking only at previous data you can treat these attributes as a looking glass into the future behavior of your customers.
In this article we’ll run through a few of our predictive attributes:
- Recommended Product
- Predicted Orders
- Predicted Spend
- Predicted Order Value
- Predicted Churn Risk.
After that, we’ll take a look at using these to create a really effective segment to target High value customers.
Recommended Product
Recommended Product pinpoints the type of product an individual is most likely to purchase next based on their past behavior. Our model analyzes historical buying patterns, preferences, and trends to identify the product category that aligns best with each customer's interests. By leveraging this data, you can tailor your marketing strategies and recommendations to match customer needs more accurately. It's a powerful tool for enhancing customer satisfaction and driving targeted marketing efforts. Combine this attribute with others, such as Last Purchase Date, to refine your segments and optimize your campaigns.
The other standard Product Recommender attributes are:
- Recommended Product Name
- Recommended Product Type
- Recommended Product Product ID
- Recommended Product SKU
- Recommended Product Size
- Recommended Product Color
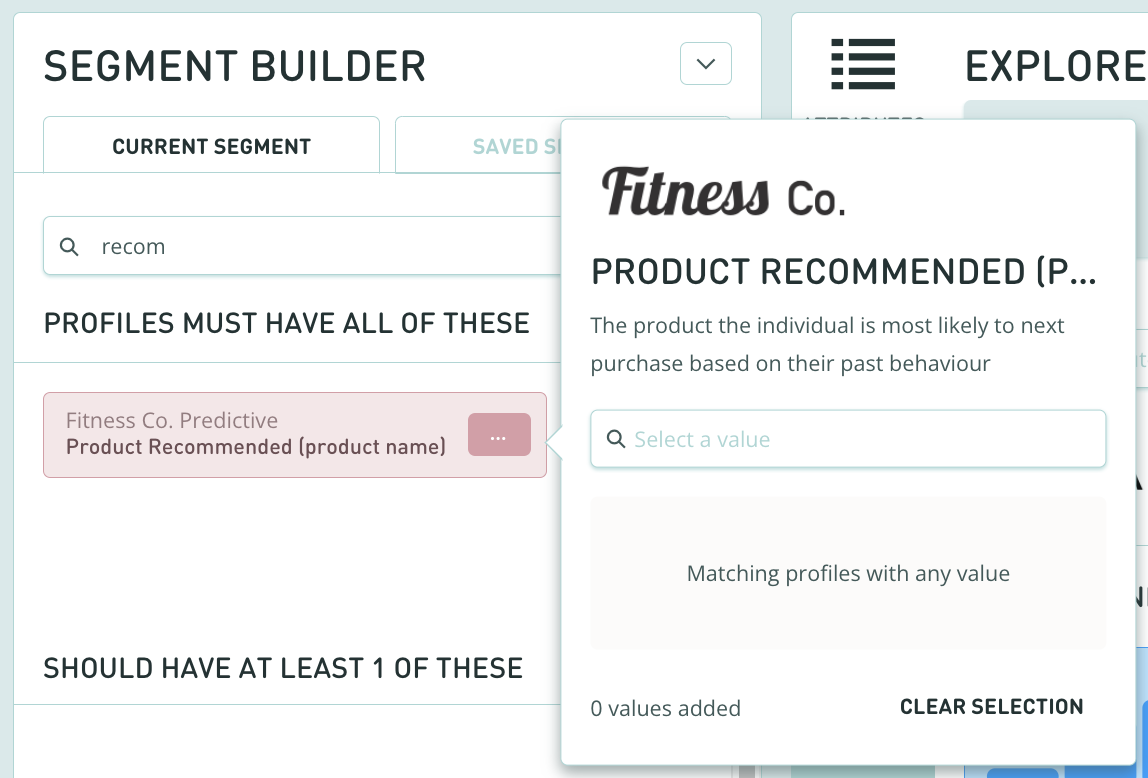
Predicted churn risk
Churn risk determines whether a customer falls into a high, medium or low risk of churn. Our model predicts future transactions using attributes such as spend per day and orders per day. It then categorizes profiles based on predicted spend, marking those below the 2nd percentile as high churn risks, 2-80 medium risk and 80-100 low risk.
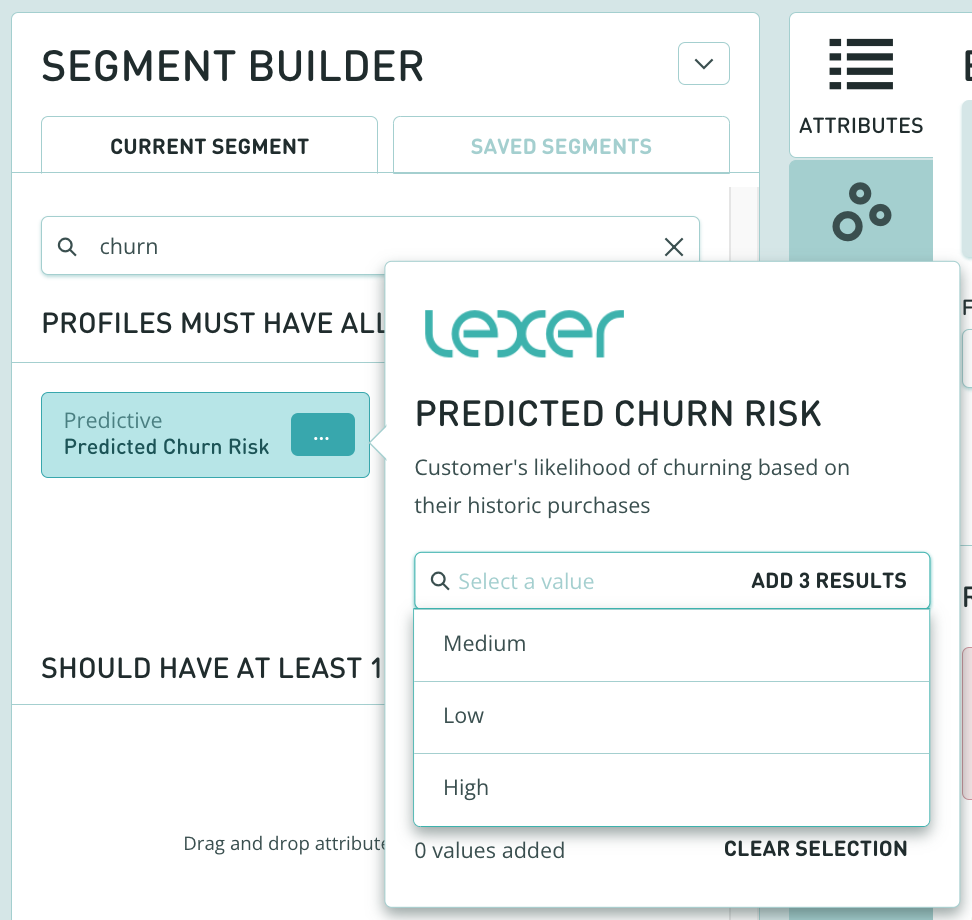
Predicted spend
Predicted spend lets you determine the parameters based on your customers spending habits to ensure this suits your businesses needs. Our model predicts the future spend of customers using daily spending, order frequency, and transaction intervals. By analyzing past data and trends, this model forecasts future spending patterns for the upcoming year. Pair this with other attributes to help narrow down your segment such as Last order date: In the last 30 days to isolate a group of customers for your next campaign. It's your ticket to staying ahead of the curve, allowing you to anticipate customer expenditure and tailor your strategies accordingly.
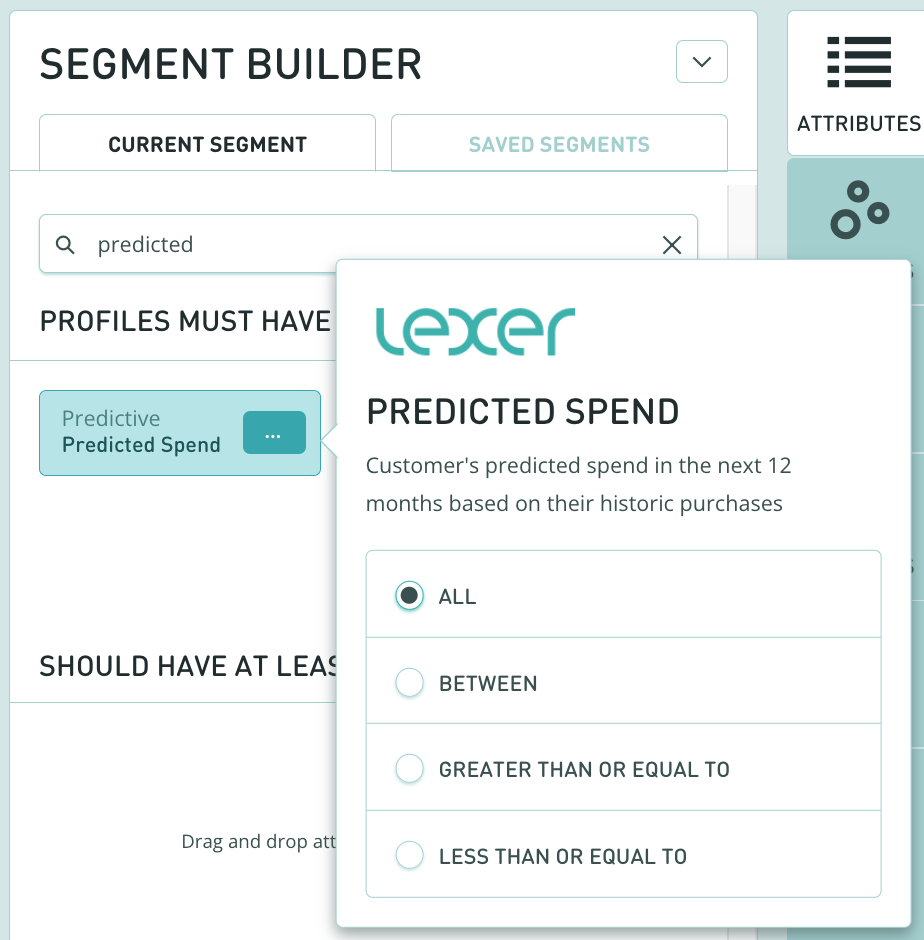
Predicted Orders
Predicted orders allows you to predict the number of orders a customer is expected to carry out in the next year. Our model predicts customers future orders using key metrics such as daily spend, order frequency, and time between transactions. By training against historical data, this model forecasts future orders and spending trends for the upcoming year. It's an awesome attribute to help you anticipate customer behavior and plan ahead with confidence.
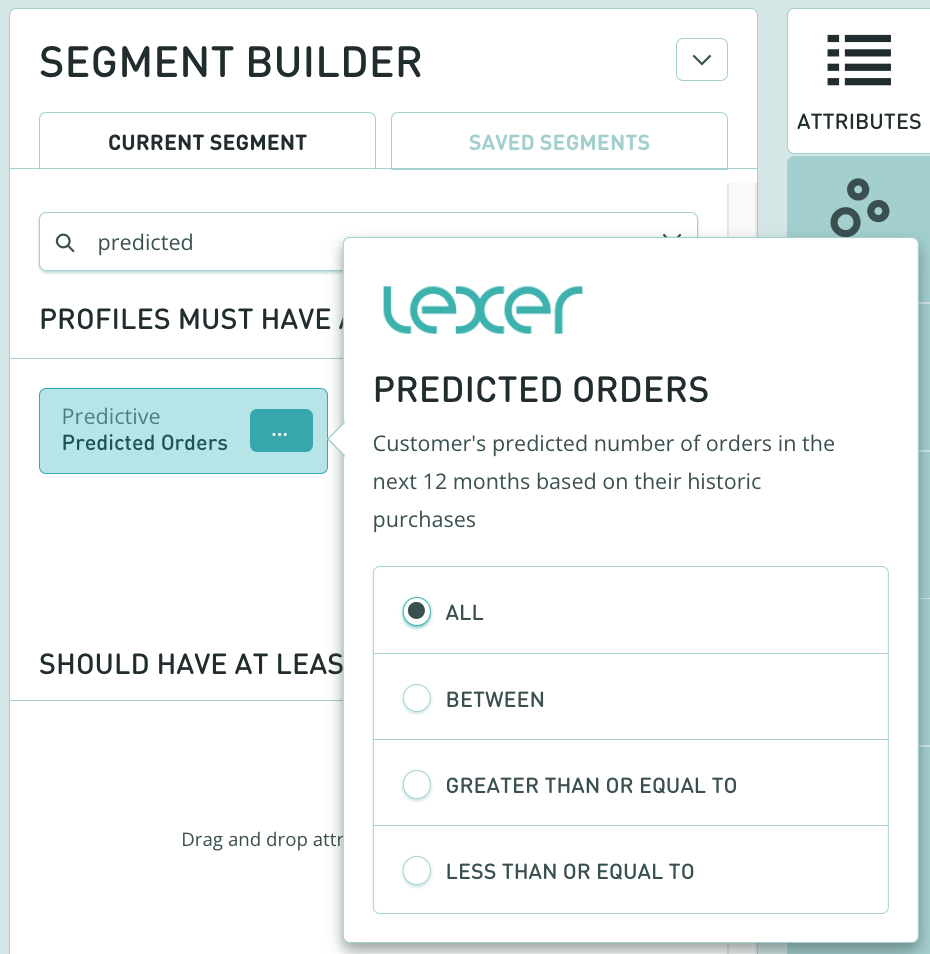
Predicted Order Value
Predicted order value allows you to predict the value of a customer's orders over the next 12 month period. This prediction uses order frequency, and time between transactions, to forecast future order values for the upcoming year. With Predicted Spend divided by Predicted Orders at its core, it provides valuable insights into customer purchasing behavior, enabling you to optimize your strategies and drive business growth.

Using predictive attributes in the Hub
Predictive attributes function like any other attribute in the Hub (see the full list here). For instance, you can add them to a segment using one of the 3 filters in the Segment builder and voila! You have a Segment built and ready to provide you all sorts of insights. For a refresher on how to build a Segment, check out our Creating segments article.
To learn more about all of the ways you can use attributes in the Hub, check out Why customers love Lexer.
Let’s take a look at a potential segment that you could build using Predictive attributes:
Fitness Co is our Athleisure inspired demo hub. We’re going to assume Fitness Co wants to create a campaign that targets consumers that are likely to spend over $150 in the next 12 months but not target any customers that might be considered a high churn risk. To create this segment, Add Predicted Spend: Greater than or equal to 150 to the Profiles must have all of these filter, and add Predicted Churn Risk: High to the But not have any of these filter.

Make sure you click Save as a new segment if you'd like to use this again.
This segment can be a really helpful tool to help you
Once you’ve built this segment, you can use it for a bunch of things in the Hub. Some examples of ways to get the most out of this Segment are:
- Send this audience a creative using your favourite ESP via Activate.
- Use Contact to send out personalised AI supported comms.
- Develop and share an Ad on your favourite socials using our Integrations.
- Interrogate your data in more detail using this segment to create more specific searches in the Segment builder.
That’s a wrap!
In this article we have covered Lexer’s predictive attributes and how they can help you create impactful segments for your business. If you have any questions, please message our Support team via the chat in the bottom right corner of the page.